It feels like with each passing year the stakes rise for predicting and managing risk. Does the universe need to keep dishing us market, environmental and health crises when regulated industries are amid massive digital transformation and analytic platform modernizations?
The insurance industry is facing down the compliance deadline for the FASB’s Long Duration Targeted Improvements (LDTI). Public carriers should be on the cusp of final transition to running new models developed over the past two years.
Similarly, most financial institutions should have their Credit Expected Credit Loss (CECL) compliance transitions in the rearview mirror. However, many credit unions have yet to reach key milestones toward implementation of the new credit standard.
And for private and smaller entities just now converting new roadmaps and processes into trial implementations, what are the lessons learned from their larger counterparts to help smooth the process?
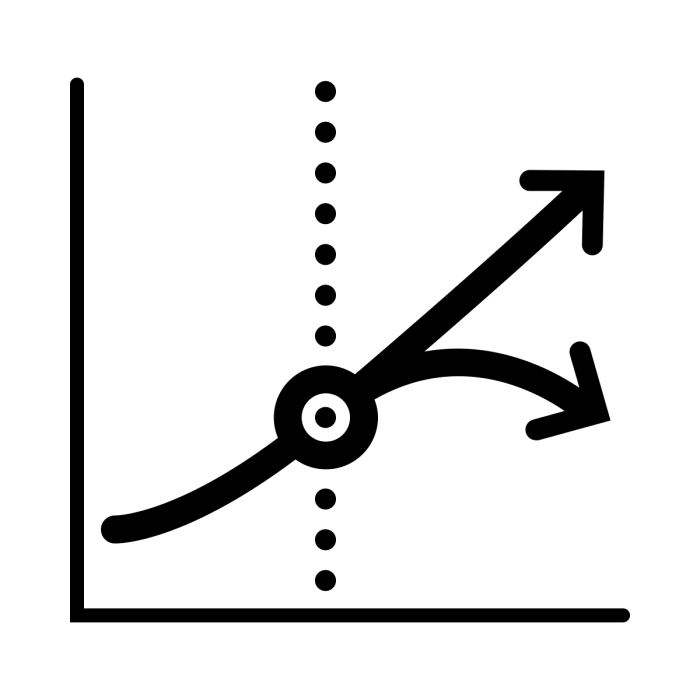
Timing, business considerations and the scope of modernization may vary among companies impacted by new regulatory standards, but there is one unavoidable similarity: all will surface analytics gaps. Deadlines can’t be missed, but issues must be addressed. All that’s left is determining how and when.